How machine learning helps predict suicide after discharge from psychiatric hospitalization
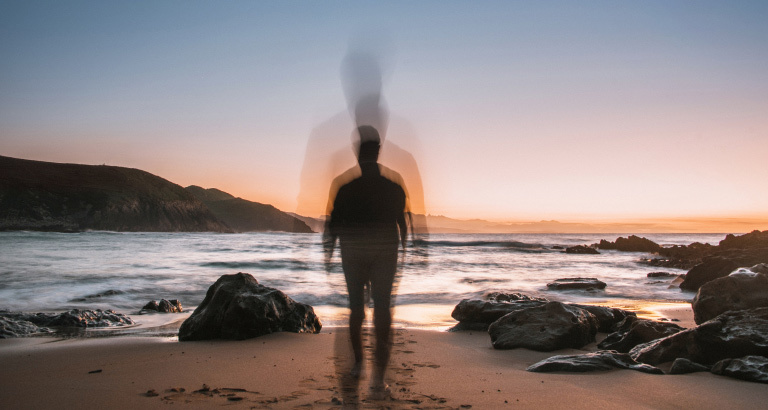
Suicide is a major public health problem, with approximately 800,000 suicide deaths globally each year. Thus, it’s crucial to accurately predict who will engage in suicidal behavior so that they may be connected with suicide prevention interventions. One key population for which suicide prevention is particularly crucial is persons who have been hospitalized for a psychiatric disorder.
The following narrative describes the burden of suicide in persons discharged from psychiatric hospitalization and what researchers at the Boston University School of Public Health have done to try to predict who is at risk of suicide within this high-risk subgroup using machine learning.
Immediately following discharge from a psychiatric hospitalization is a high-risk period for suicide
Suicide rates are particularly high immediately following discharge from psychiatric hospitalization. The suicide rate among persons in the first month after discharge from a psychiatric hospitalization is over 190 times the global suicide rate. This shows that the immediate period after leaving inpatient psychiatric care is an essential window of opportunity for prevention practices to be set in place to reduce suicide deaths.
A key question is who is at the highest risk of suicide after discharge and may benefit from suicide prevention interventions?
Our team at the Boston University School of Public Health and Aarhus University in Denmark sought to examine this question and recently published our findings in the British Journal of Psychiatry.
How machine learning helps identify people at high risk of suicide after discharge
We used machine learning methods and Danish registry data to predict suicide deaths in the 30 days following discharge from psychiatric hospitalization. Machine learning methods are useful for research problems that involve predicting health outcomes from a large set of variables with complex interactions and relationships. This helped us identify complex patterns in the data that are informative for identifying combinations of characteristics associated with suicide.
In men, we found that prescriptions for psychiatric medications such as anti-anxiety drugs, drugs used in addictive disorders, and hypnotics and sedatives, and diagnoses of alcohol-related disorders were associated with a high risk of post-discharge suicide.
In women, we found that recurrent major depression, prior poisoning, and low income were associated with a high risk of post-discharge suicide.
The sex-stratified models showed that persons in the top 20% of predicted risk accounted for 59% of suicide cases in men and 71% of suicide cases in women.
Conclusion
The immediate period after discharge from a psychiatric hospitalization represents a high-risk period for suicide. Using machine learning, we can identify persons likely to make a fatal suicide attempt in this vulnerable population. Accurate prediction of suicide requires evaluating numerous psychiatric, medical, and social risk factors. This study has helped us pinpoint a high-risk factor for suicide, and hope this and future research will aid in further understanding suicide risk.
If you are experiencing suicidal thoughts of any kind and are in need of someone to talk to, don’t hesitate to reach out to the following resources:
Dr. Tammy Jiang is a postdoctoral research scientist in the epidemiology department at the Boston University School of Public Health. Her research focus is in psychiatric epidemiology, and her work applies advanced epidemiologic methods with machine learning approaches to analyze mental health and suicide outcomes.