Promising data for prediction of post-disaster mental health needs in an evolving disaster landscape
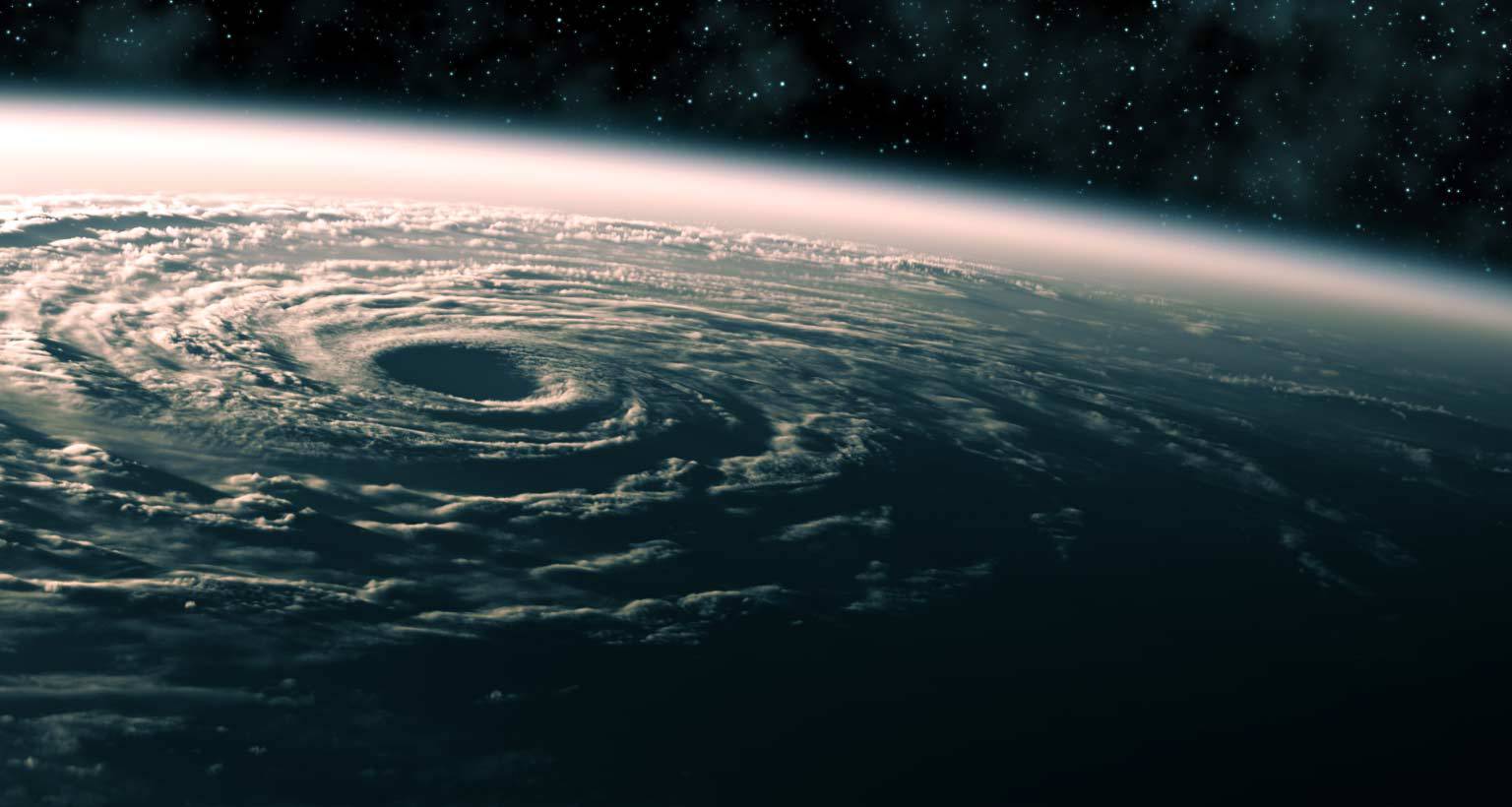
There is no question that the disaster landscape is becoming more dangerous and complex. Our warming global climate has steadily brought more intense hurricane seasons and increasingly dangerous heatwaves and fires. COVID-19, which converged with wildfires on the West Coast and the Atlantic hurricane season in the Gulf Coast, has taxed our disaster response system and presented new challenges. This intensifying disaster landscape calls for more rapid, comprehensive, and efficient ways to accurately predict the mental health needs of disaster survivors so that we may effectively triage resources to address those needs.
The pros and cons of self-reported surveys
Surveys are perhaps the most common methodology for the identification of mental health services need. Screening for mental health symptoms can be done in health care settings or shelters, or can even involve fielding a geographically focused random probability sample. Screening for mental health risk factors to identify the mental health needs of a particular population is another approach. For example, the PsySTART system created in collaboration between UC Irvine, American Red Cross, and Stony Brook, primarily screens for exposures that predict mental need, such as disaster-related physical injury, being trapped, or having a delayed evacuation, or having a family member currently missing.
Risk factors in the PsySTART system
- Extreme panic/fear
- Felt life threat
- Saw/heard injury or death
- Family member death
- Pet death
- Disaster-related physical injury
- Trapped/delayed evacuation
- Family member currently missing
- Child currently unaccompanied
- Home not livable
- Family separated during event
- Mental health history
- Disaster/trauma history
- No risk factors observed
Acute Assessment Required
- Danger to self or others
These questions have some advantages to psychiatric symptom screeners in that they avoid the stigma that is often associated with mental health symptoms, and also that they may identify symptom risk prior to the emergence of symptoms. But while self-reported surveys are often some of the best data available, they are limited by those who present for services or care, and by those who participate in surveys. Those who don’t present to care or participate in surveys cannot contribute to these data, and we are left with an incomplete picture.
The question then emerges—What other data can be used to augment this self-reported data to predict the need for mental health services following disaster exposure?
New data sources
There are five additional data sources that may productively serve as foci:
- passive satellite imaging
- social media
- Google searches
- prescription monitoring
- area deprivation risk indices
In our current era of big data, machine learning, and complex systems modeling, there is indeed much room to augment standard methods of predicting population mental health needs using such data.
- Passive satellite imaging data was employed following Hurricane Sandy as a key part of FEMA’s exposure mapping processes, along with field-based data collection on storm damage. These area-based data indeed appear to predict PTSD risk somewhat well in a population of Hurricane Sandy survivors. While there is much room to refine this type of prediction, including population level-validation, these data clearly hold promise and are amenable to the incorporation of machine learning techniques.
- Social media data, Twitter, in particular, appears to have great potential in identifying mental health services needs, when combined with machine learning algorithms. A study by Reece and colleagues through Harvard University, University of Vermont, and Stanford University, showed that their computational approaches to identifying mental health need using Twitter content was able to discriminate reasonably well between cases and non-cases of both depression and PTSD. This approach could be quite effective in identifying hotspots of mental health need, and could be easily and productively applied to delineate geographic hotspots of mental health need over time. Notably, it will be critical to find the best area-level (i.e., zip-code vs. census block vs. census tract), and time scale at which to measure and track population mental health needs.
- Google search data is another promising and emergent source of information that may be harnessed to identify mental health needs following population mental health shocks such as extreme flooding or tornados. Recent research showed a rise in Google searches for “anxiety” or “panic” in combination with the word “attacks” following the declaration in March, of a national COVID-19 emergency in the United States. As shown in figure 2, the data appears to pick up the expected upward tick in those searches over the course of March and April. While there is much to be learned about the utility and measurement characteristics of these data, and the degree to which they may reflect normative general distress rather than conditions of clinical concern, they have much potential.
- Prescription monitoring data systems are a fourth set of data that may help optimize our identification of emergent mental health services need. For example, following the deadly 2010 Xynthia windstorm, which took place in Western France, the National Health Insurance database was used to examine trends in new prescriptions of tranquilizers, hypnotics, and antidepressants within the disaster-affected area. As shown in figure 3, they observed a spike in new prescriptions in the first three weeks following the storm. Similar work has also been done in England using interrupted time series methods, to examine regional effects of flooding events on antidepressant drug use. This approach presents particular challenges in the United States, given the fragmentation of health care systems, but may be possible nonetheless.
- Area deprivation risk indices represent a fifth set of data that may help in augmenting post-disaster identification of mental health services need. While there are numerous examples of scholarship demonstrating the influence of socioeconomic forces on mental health at the individual and community levels, I am not aware of any work that employs a socioeconomic risk scoring approach to area prediction of mental health services need. The advantage of such an index is the ability to simultaneously consider numerous key factors that predict services need. Whereas census-based area level deprivation indices have been used to predict life expectancy and suicide risk, they have not been used to aid in the prediction of post-traumatic stress and depression. The CDC has developed a Social Vulnerability Index with 15 census-based area indicators (e.g. poverty, crowding, lack of vehicle access), that can be used at varying geographies, including the census tract level, as shown in figure 4 – a map of Suffolk County, which includes the city of Boston. Such data will likely be most useful when layered with maps of disaster exposure.
Piecing together the data puzzle after a disaster
While none of these data sources can alone accurately determine mental health services need, together, they may be able to help augment standard survey approaches. Each may have their own limitations, but also fills out a different piece of the data puzzle. Many challenges to improving area prediction of mental health services remain, including small area estimation, and finding appropriate validation opportunities, but there is little doubt that these data would help.
The primary question moving forward then is how to integrate these disparate data sources into a unified prediction model?
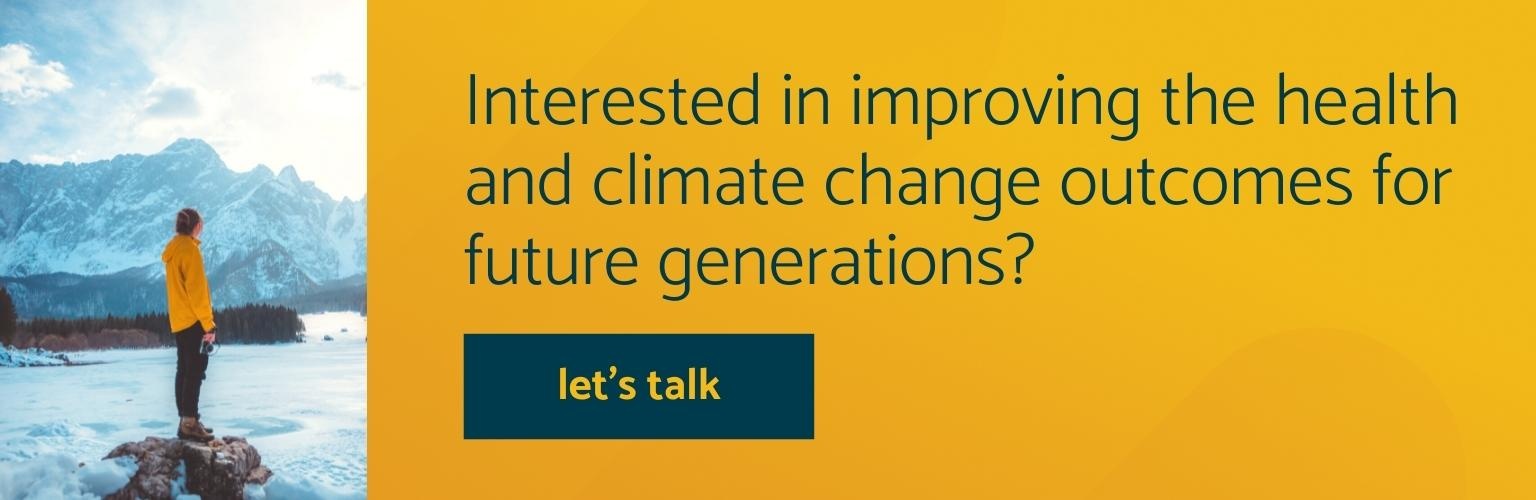
Dr. Gregory Cohen, is a Postdoctoral Fellow in the Department of Epidemiology at Boston University School of Public Health. His main research interests include the mental health consequences of trauma, social disparities in mental health and chronic disease, and equity-efficiency tradeoffs in population health interventions.