Communicating and promoting replicability in science
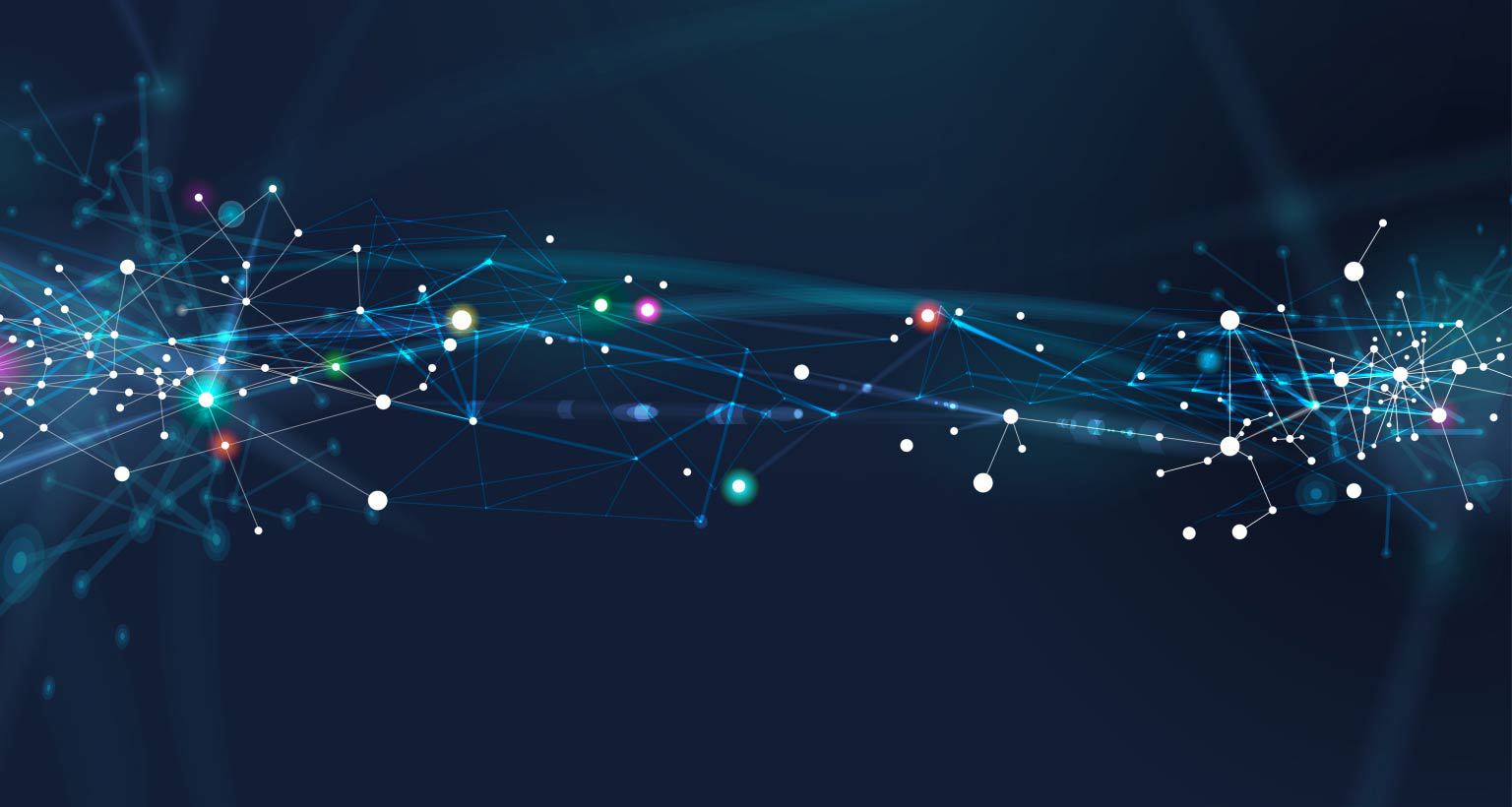
Scientific research is facing a reckoning in the court of public opinion. Anti-science sentiment has manifested in a lack of trust in institutions, claims of frivolity leading to cuts in funding, and general vitriol for what are perceived to be elitists making unilateral, unpopular decrees from ivory towers. In large part, the scientific community has not taken these issues lightly and is undergoing substantial introspection to study (as we do) the root causes of what has germinated into widespread distrust.
The replicability in science debate
One such hot-button issue both within and without the scientific community is a lack of reproducibility and replicability in science. Many large-scale efforts to repeat previously published experiments and confirm their results and conclusions have come up alarmingly short, prompting serious questions about the peer review process and whether the incentives for academic publication are indeed aligned with the goals of scientific discovery [1]. These efforts have incited raucous discussion about the pervasiveness of irreplicable research practices and short-term and long-term solutions. Conversations like these are of special interest to biostatisticians like me because the comparison points between an original finding and a replication finding often boil down to statistical tests or comparisons of significance/p-values.
There are two general topics that have stood out to me as I have watched the “replicability crisis” unfold. First, as evidenced by the various words for the phenomenon that I purposely used in the previous paragraph (reproducibility, replicability, repetition), specific definitions and terminology have not been universally established for this discussion. Different disciplines of science can have wildly varying definitions for the same terms, and we have not done a good job of establishing what we mean when we say “replication” in the company of colleagues who may be operating from a completely different conceptual base.
This confusion affects our expectations for what should happen when we try to replicate an experiment as well as how we measure and compare the replicated experimental result against the original result. Second, although a number of fixes related to more stringent thresholds for significance and pre-registration of experimental design have been proposed, there has not been a widespread call to incorporate replicability as a direct objective in the conduct of research.
Personally, I like to define reproducibility and replicability in terms of an idealized academic publication, in which the authors have clearly laid out and made publicly available all pertinent aspects of their study:
- their question of interest and hypothesis
- experimental design and data collected
- analytic plan and code to conduct the analysis
- their final results and conclusions
In this context, I define reproducibility as “if I take your data and apply your code, do I obtain the exact results that you reported?” In contrast, I define replicability as “if I take your experimental design and analytic plan and use them to collect and analyze a new data set, will I obtain results that are in agreement with your reported findings?” Note that these definitions differ in the level of evidence they require. For example, if you studied a new drug therapy and found that it was 10% more effective than a placebo, my reproduction of your work (using your published data and code) should yield exactly 10% improvement, while my replication of your work (using new data and code based on your designs) does not necessarily have to report 10% improvement for our two studies to agree. I prefer these definitions because they differentiate the two terms to reduce confusion and allow us to focus on what level of “agreement” is necessary to claim that a study has been replicated.
Visualizing the differences in reproducibility and replicability
As all researchers do not ascribe to the same definitions, we have created an R package called scifigure which creates simple visualizations so that any definition for any such related term can be conveyed in the context of the steps of a generic study. As an example in Figure 1, definitions for reproducibility and replicability are visualized. For reproducibility, only the analyst (colored in blue) changes from the original to the reproduction effort, as a new analyst would use the original data and code to get the same exact results (estimates and claims). For replicability, the experimenter and the data they collect changes from the original to the replication, along with the analyst, code used to analyze the data, and the eventual results. The question there is whether or not the original estimate and claim are in agreement with the findings in the replication.
Specific problems in replicability
The preceding refers more to the question of scientific replicability as an aspect of general practice, but replicability issues also manifest within specific problems in science. One such issue is why some predictive models do not generalize well to different patient populations. Consider the case of developing a model that uses gene expression information to categorize ovarian cancer patients into risk groups which indicate how likely they are to suffer a future recurrence of cancer. If a patient is deemed to be at a high risk for future recurrence based on the prediction from this model, their doctor may elect for a more aggressive form of therapy today.
Unfortunately, many of the models of this type proposed in the literature never make it out of the literature and into regulatory approval to become a clinical test. If they do, the test is often only applicable to a subset of all patients that exhibit certain characteristics, and these characteristics are often in line with the original patient cohort upon which the predictor was trained. This is a problem because it is possible that the originally published predictor is overfit to the original dataset, meaning that the model picked up on patterns that are specific to the particular sample of patients which do not constitute a general relationship between gene expression activity and risk of cancer recurrence. As a result, the predictor may not have performed as well in patient cohorts gathered at other hospitals, who may have had their gene expression measured using different technologies, or who may otherwise vary in clinical characteristics or selection criteria. Once again, irreplicability has reared its ugly head.
In the past, it was a challenge to validate these gene expression prediction models because it was costly to collect a single patient cohort upon which to train the model, and access to external patient cohorts was limited. As data storage and latency has improved, we now have far more access to multiple patient cohorts. A major question of interest is whether we can integrate multiple patient cohorts to train a predictor that would perform better on a new set of patients than a predictor we could have trained using any one of the individual cohorts. One strategy would be to combine all of the datasets together and train a single predictor on all available patients, and while this greatly increases the sample size of the training set, it ignores the inherent cohort-to-cohort heterogeneity that is one primary source of irreplicable behavior.
A different approach to cohorts
We have championed an ensembling approach as an alternative: supposing we have 10 different patient cohorts, we train one predictor on each cohort. To make a prediction on a new observation, we collect the predictions from each of the 10 predictors and compute their average. To promote replicability directly, we can assign weights to the contributions of each of the 10 predictors that reflect how well each one generalizes to other training studies. Using an existing set of predictors published for ovarian cancer risk prediction, we have demonstrated that this ensembling approach can improve predictor performance on an external cohort above and beyond the performance of the best single predictor [3]. Although this is just one of many possible strategies, we believe that it is the specific promotion of replicable behavior using the ensembling weights that makes this approach successful.
Replicability issues in science manifest both in how we communicate our findings and in how we conduct our research. The past few years have greatly increased our awareness of these issues, and there are promising solutions being proposed at all levels to increase the replicability of our work. I believe that as long as we ensure that the problem is laid out clearly and in unambiguous terms, and that we make replicability an objective of research practice, we will be well on our way toward regaining the public’s confidence and reestablishing the foundation of trust upon which the scientific institution is built.
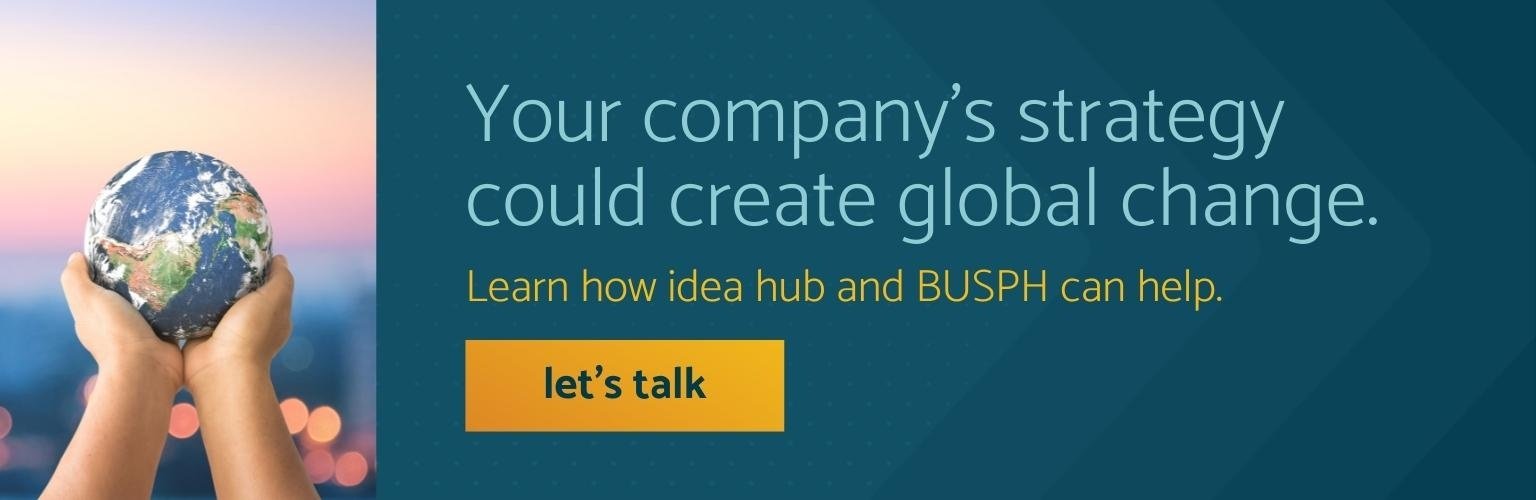
Dr. Prasad Patil is an Assistant Professor of Biostatistics at the Boston University School of Public Health. His professional interests include personalized medicine, genomics, machine learning, data visualization, and study reproducibility/replicability. Dr. Patil is currently working on multi-study prediction statistical definitions for reproducibility and replicability as well as replicable and interpretable methods for training genomic biomarkers.
References
- Patil P, Peng RD, Leek JT. A visual tool for defining reproducibility and replicability. Nature human behaviour. 2019 Jul;3(7):650-2.
- Patil P, Parmigiani G. Training replicable predictors in multiple studies. Proceedings of the National Academy of Sciences. 2018 Mar 13;115(11):2578-83.